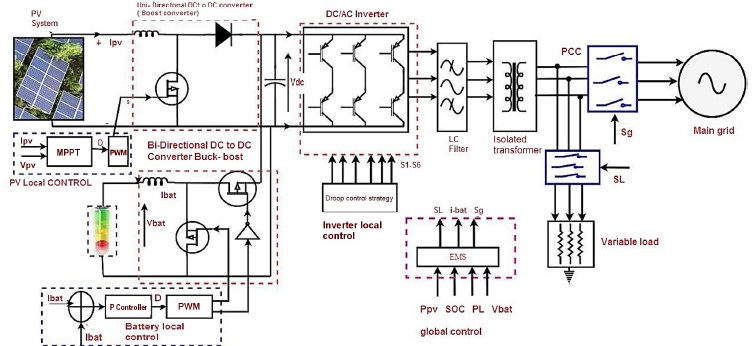
Received 14 Jul. 2023
Received in revised form 27 Sep. 2023
Accepted 29 Sep. 2023
Available on-line 08 Nov. 2023
Keywords: Renewable energy; photovoltaic; grid-connected system; energy management system; control strategy; disturbances;
Growing energy demands are expected to render existing energy resources insufficient. Solar energy faces challenges in terms of providing continuous and reliable power supply to consumers. However, it has become increasingly important to implement renewable energy (RE) and energy management (EM) systems to increase the supply of power, improve efficiency, and maintain the stability of energy systems. As such, this present study integrated energy storage (ES) devices; namely, batteries and direct current (DC) to DC converters; into energy systems that support battery operation and effectively manage power flow, especially during peak load demands. The proposed system also addresses low solar irradiation and sudden load change scenarios by enabling the battery to operate in a discharge state to supply power to the load. Conversely, when the demand matches or exceeds the available solar energy, the battery is charged using solar power. The proposed system highlights the significance of RE systems and EM strategies in meeting growing energy demands and ensuring a reliable supply of power during solar variability and fluctuating loads. A MATLAB® Simulink model was used to evaluate the integration of a 200 kW photovoltaic (PV) array with a 380 V grid and 150 kW battery. The loads, consisting of a 100 kW and a 150 kW unit, were parallel connected. The results indicated that boost and three-phase (3Ph) inverters can be used to successfully integrate PV systems to the power grid to supply alternating current (AC) power. The inclusion of a battery also addressed power shortages during periods of insufficient power generation and to store surplus power.
The total global energy derived from renewable energy (RE) sources is estimated to exceed 4 GW by the end of 2023 [1]. Photovoltaic (PV) energy is a significant source of RE and accounts for approximately 47% of all newly installed RE generation systems [2]. In order to increase the use of novel technologies in the form of RE sources, like wind turbines, photovoltaics (PV), and biomass, which are regarded as sustainable and eco-friendly with zero fuel costs and easy access, many nations have changed their policies pertaining to the generation of electricity. PVbased renew-able systems can operate in both stand-alone and grid-connected modes. The production, control, and communication units are the major components of a microgrid. The optimum usage of various energy sources may yield economic and environmental advantages [3]. Microgrids also prioritize network efficiency, financial feasibility, power distribution, and long-term energy storage (ES). It may comprise several or one single linked component that relies on alternating current (AC), hybrid, or direct current (DC) links as the common connection point (CCP) [4]. Multiple studies have examined energy management (EM) systems, as well as numerous control methods and controllers to create energy units [5–9]. The EM system is considered a top-level control system for supervisory control and data acquisition [10–14].
RE systems are designed to operate within certain limits in normal circumstances. However, these systems are susceptible to power distortions with varying effects, durations, and intensities that can impact their perfor-mance. One notable challenge with hybrid grid-connected systems is EM using an EM system. EM systems handle a variety of tasks, like effectually charging and discharging the storage battery from the grid or PV plant setups. By supplying customers with high-quality, uninterrupted power during disruptions, this mechanism plays a crucial part in guaranteeing the safety and effectiveness of power systems. To overcome these issues, control methodologies, such as converters that ascertain deviations between measured values and reference values, are used. The current, voltage and the amount of power delivered can all deviate from the norm. Therefore, these control techniques main goal is to improve hybrid systems performance in the presence of disruptions. One example is maintaining a balance between the amount of power generated and the load demanded. When the load demand is light, the excess energy generated by a PV system is stored in a battery. However, when power distortions occur and the load demand abruptly exceeds the power generation capacity of the PV system, the battery discharges the stored energy to ensure system stability within acceptable limits. Many research works have, in recent years, concentrated on the EM capabilities of such setups under normal, as well as abnormal situations, thanks to the improvements in RE systems and the growing global tendency towards hybrid grid-connected systems, given their aforementioned bene-fits. The behaviour of hybrid systems under different forms of disturbances is covered in this section through a few quick summaries of key studies that discuss how to optimise the performance of hybrid systems and ensure their resilience under challenging circumstances. Refs. 15–17 used multi-objective or stochastic optimisation methods to optimise EM in uncertain energy shift scenarios. Mean-while, Ref. 18 proposed a control strategy and EM system with a managerial control that takes into account the difference between real-time and off-line schedules to analyse the grid-connected and islanded modes. The proposed method used low-level decentralised control to control the local distribution generator and high-level centralised control to address bidirectional (BD) power sharing and communication issues. The ideal dispatching method for a medium voltage islanded microgrid with a storage setup is a heuristic evolutionary algorithm. While Ref. 19 thought about operation scenarios for zone-based multiple microgrids, Ref. 20 provided a comparison and substantiation of a real microgrid. Ref. 21 explored decen-tralised EM systems and intelligent controls for autonomous poly-generation microgrids, and the algorithms used fuzzy cognitive maps to create the desalination system. Decen-tralised control was used in microgrids powered by PV cells and battery storage, and the results were only displayed virtually. In order to demonstrate the efficacy of hierarchical EM models for distributed energy resources (DER) [22, 23], advanced optimisation techniques such as neural networks, swarm optimisation, and sequential quadratic programming were used in simulations or a hardwire in the loop (HIL) setting. Ref. 24 investigated the use of a static synchronous compensator (STATCOM) along with a decentralised control approach for a hybrid AC-DC microgrid. To substantiate the recommended strategy superiority, it was contrasted with the droop controller-based approach. A standardised approach for comparing the relative cost-effectiveness of microgrids propelled by lead-acid and lithium-ion batteries was presented by Ref. 25. Utilising lithium-ion batteries appears to be 6 to 7% more lucrative when grid power costs, state of charge (SOC), and power balancing modes in EM systems are considered.
This present study aimed to examine and analyse the behaviour and performance of a battery-PV hybrid grid-connected system during disruptions, such as abrupt load changes and rapidly changing levels of solar irradiation. The microgrid and the potential power disruptions were simulated in MATLAB® Simulink with a 200 kW PV array linked to a 380 V grid. Three distinct control strategies were introduced: namely, maximum power point tracking (MPPT) for the boost converter, pulse width modulation (PWM) for the ES system, and PWM for the three-phase (3Ph) inverter. The primary objective was to assess how effectively these strategies uphold system performance and stability in the face of power disruptions.
As seen in Fig. 1, the microgrid was configured as a PV system linked to a DC link by a boost-based unidirectional (UD) converter and comprised an inverter with two levels linked to an inductor and capacitor (LC) filter, a battery storage setup linked to a buck and boost-based BD converter to maintain system stability in various modes, and a variable load for the test system linked at the point of common coupling (PCC). The entire setup was linked to the main grid by an inverter. Equations (1) and (2) yield the PV systems and battery power.
\[ \begin{gathered} P_{p v}=V_{p v} I_{p v} \end{gathered} \] (1)
\[ P_{b a t}=V_{b a t} I_{b a t} \] (2)
where 𝑉𝑝𝑣 , 𝐼𝑝𝑣 are the PV voltage and current, while 𝑉𝑏𝑎𝑡,
𝐼𝑏𝑎𝑡 are the battery voltage and current.
The voltage-current characteristics of the battery
system can be expressed as
\[ P_{\text {bat }}=V_0-\mathrm{K} \frac{C_b}{C_b-\int I_{\text {bat }} d t}+A \cdot e^{\left(-\int I_{\text {bat }} d t\right)}-R_b \] (3)
where 𝑅𝑏 and 𝐶𝑏 are the battery internal resistance and maximum capacity, respectively, 𝜏 is the time constant,
𝐴 is the exponential area amplitude, and ∫ 𝐼𝑏𝑎𝑡𝑑𝑡 is the capacity of the battery. Equation (4) was used to calculate the required SOC, which was used to govern the battery storage systems discharge and charge cycles.
To preserve the life cycle of the battery, the SOC should be maintained within the 𝑆𝑂𝐶 𝑚𝑖𝑛 and 𝑆𝑂𝐶 𝑚𝑎𝑥 which are, based on the battery characteristics, its lowest and highest allowable limits. The eventual formula for the active (𝑃) and reactive (𝑄) output power in 𝑑𝑞0 with 𝑣𝑑 = 𝑉, 𝑣𝑞 = 0 is as follows:
\[ \begin{aligned} P=3 v_d i_d \end{aligned} \] (4)
\[ \begin{aligned} Q=3 v_d i_q \end{aligned} \] (5)
where 𝑖𝑑 𝑎𝑛𝑑 𝑖𝑞 are the inverter output currents.
Equation (6) was used to balance the power and meet the load demanded when in the grid-connected mode.
\[ P_g=\left(P_{P_v}+P_{\text {bat }}\right)-\mid P_L-P_{\text {LoSSES }} \] (6)
where 𝑃𝑔 and 𝑃𝑃𝐿 are the grid and load powers, respectively, 𝑃𝑝𝑣 and 𝑃𝑏𝑎𝑡 are the PV and battery powers, respectively, and 𝑃𝐿𝑜𝑠𝑠𝑒𝑠 is the power losses of the LC filter. Equation (6) was used in the power management algorithm to supervise the whole system and maintain it in the balance mode. Table 1 provides the detailed specifications of the proposed PV system.
Table 1.
PV array specifications
Parameters |
Value |
No. of series panels that can be connected |
9 |
No. of parallel panels that can be connected |
58 |
Maximum power of panel |
400.2 W |
Open circuit voltage of PV system |
768 V |
Rated current of the PV system |
312.9 A |
Rated voltage |
656 V |
Total rated power |
205 kW |
Total short circuit current |
334.5 A |
The amount of energy output by solar systems can be optimised using MPPT. Of many methods of achieving the maximum output power, this present study used the perturb-and-observe (P&O) algorithm [26]. The maximum power point (MPP) may be determined by varying the duty cycle of the boost converter and comparing the prior and current levels of power (𝑃) and voltage (𝑉). When the Δ𝑃/Δ𝑉 is negative, the operating point is on the right side of MPP and vice versa. A boost-based UD converter was used to increase the DC voltage from 656 V to 800 V. The converter was able to get the most out of the PV system by adjusting the duty cycle.
This present study also used a capacitance filter to filter the output voltage and mitigate high-current circuit losses. Equations (7) and (8) specify the inductor and the output capacitor of the boost converter
\[ \begin{aligned} L_{\min } & =\frac{D(1-D)^2 R}{2 f} \end{aligned} \] (7)
\[ \begin{aligned} C & =\frac{D}{R\left(\frac{\Delta V_o}{V_o}\right) f} \end{aligned} \] (8)
where ( Δ𝑉o / 𝑉o ) is the output ripple voltage, 𝑅 is the output resistance, and 𝑓 is the frequency [27]. The resistance of the output capacitor and inductor were assumed. Table 2 lists the boost converter settings utilised. Table 2.
Boost converter parameters, at duty cycle 𝐷 = 0.5 and switching frequency = 4 kHz.
Parameter |
Value |
Parameter |
Value |
Input voltage |
656 V |
Output voltage |
800 V |
Inductor |
0.68 mH |
Output capacitor |
2.4 mF |
A DC link capacitor was used to decrease the voltage variance of the DC connection between the power converters, as well as maintain a continuous supply of power to the inverter. Equation (9) was used to calculate the rootmean- square (RMS) of the capacitor ripple current.
\[ I_{\text {cap,r.m.s }}=I_{N, r . m \cdot s} \sqrt{\left[2 m\left\{\frac{\sqrt{3}}{4 \pi}+\theta\left(\frac{\sqrt{3}}{4 \pi}-\frac{9}{16} m\right)\right\}\right]} \] (9)
where 𝐼𝑁,𝑟.𝑚.𝑠 is the inverter output phase current which was delayed with a 𝜃 phase delay, and 𝑚 is the modulation index [28]. The minimum capacitance value was calculated as follows:
\[ C_{\text {dc-link }}=\frac{I_{\text {cap,r.m.s }}}{w_s \cdot V_{\text {ripple }}} \] (10)
The DC-link capacitor is equal to 14.8 mF, when 𝑚 = 0.6, 𝜃 = 0, 𝑉𝑟𝑖𝑝𝑝𝑙𝑒 = 1%, 𝑤𝑠 = 2𝜋 · 4 kHz and the power transfer through the inverter is 200 kW.
A 3Ph voltage source inverter block was locked into the correct operating frequency to keep the frequency stable and manage the voltage and frequency, balance and level the load, and decrease the amount of total harmonic distortion (THD) that occurred. Fig. 2 depicts the control strategy that was used to concurrently accomplish all the above-mentioned objectives in both the grid and islanded operation modes and maintain a constant output voltage during changes in the amount of power generated or load power profile.
The 3Ph voltages and currents were measured and converted into two components, 𝑑 and 𝑞, then compared with reference values. They were then applied to a proportional integral (PI) and a PWM controller to control the input signal of the inverter [28]. A BD buck- and boostbased converter [29] has immense potential for efficient energy transfer as both switches play a pivotal role in directing the flow of power. The metal-oxide-semiconductor field-effect transistor (MOSFET) (1) switch functions as a buck converter and channels power to the ES battery, as well as ensures the optimal utilisation and preservation of power while the MOSFET (2) switch kicks in when power must be channelled to the grid, thereby, facilitating the seamless exchange of energy.
\[ L=\frac{V_{\text {inmin }}\left(V_{\text {inmax }}-V_{\text {out }}\right)}{f_s \cdot \Delta I L_{\text {max }}} \] (11)
where 𝑉𝑖𝑛𝑚𝑖𝑛 and 𝑉𝑖𝑛𝑚𝑎𝑥 are the battery voltage (𝑉𝑏𝑎𝑡) and the DC bus voltage (𝑉𝐷𝐶_𝑏𝑢𝑠) or vice versa, 𝑓𝑠 is the switching frequency, and Δ𝐼𝐿𝑚𝑎𝑥 is the maximum allowable change in inductor current. For example, if the input 𝑉𝑏𝑎𝑡 is 740 V and the output 𝑉𝐷𝐶_𝑏𝑢𝑠 is 800 V, the ripple of the output and input current is assumed at 1% and the switching frequency is 4 kHz, the suitable inductor current is 3.7 mH.
The BD converter was controlled by comparing the deviations of the DC bus voltage and battery current with their respective reference values. Two PI controllers were combined with PWM to generate pulses for the two switches. The BD converters comprised buck and boost converters. The buck converter operated when the amount of PV-generated power available exceeded the power load demanded and the boost converter operated when the power load demanded exceeded the amount of PV-generated power available. This control strategy also accounted for the battery voltage and SOC during operation. Variations in the voltage of the battery can also be referred to as changes in the operation modes [30].
The primary function of an LC filter is to decrease the THD of the waveforms of the generated currents and voltages as they may cause losses and distortions in the output power. They are commonly used as they are both simple to use and inexpensive. Empirically, the LC filter can be set at induction (𝐿𝑓 ) = 0.25 mH and capacitance (𝐶𝑓 ) = 46 μF.
The flow chart as shown in Fig. 3 provides a structured approach to optimizing the use of renewable energy sources, balancing grid interaction, and managing battery operation to ensure efficient and reliable power supply. It serves as a foundation for implementing control strategies in real-world RE systems. The primary purpose of the proposed EM system is to efficiently balance the generation of electricity from the PV panels, the demand for electricity by loads, and the SOC of the battery to optimize energy utilization. It has many points of view:
Data collection: the procedure starts by collecting real-time data, including PV power generation (𝑃𝑃𝑉 ), battery SOC, load power demand (𝑃𝑙𝑜𝑎𝑑 ), and battery voltage (𝑉 𝑏𝑎𝑡 ).
Load and PV generation forecasting: load demand is forecasted (𝑃𝑙𝑜𝑎𝑑 −𝑝𝑟𝑒𝑑 ), and PV power generation is estimated (𝑃𝑃𝑉 −𝑝𝑟𝑒𝑑 ) to anticipate future energy requirements.
Mode selection: based on real-time data and forecasts, the code selects an operating mode:
Grid-connected mode: when PV power exceeds the load demand, surplus energy can be injected into the grid (𝑃𝑖𝑛𝑗𝑒𝑐𝑡𝑒𝑑 ), or power can be drawn from the grid (𝑃𝑔𝑟𝑖𝑑 ) if load demand exceeds PV generation.
Battery charging mode: if PV generation exceeds load demand and the battery SOC is below a minimum threshold (𝑆𝑂𝐶𝑚𝑖𝑛 ), the excess energy is used to charge the battery.
Battery discharging mode: when PV generation is less than load demand and the battery SOC is above a maximum threshold (𝑆𝑂𝐶𝑚𝑎𝑥 ), the battery discharges to supplement the load.
Islanded mode: in this mode, the code ensures that PV generation is utilized to meet the load demand while considering battery discharge limits.
Battery operation management: depending on whether the battery is in charging or discharging mode, the code calculates the appropriate power level (𝑃𝑏𝑎𝑡𝑡𝑒𝑟𝑦 −𝑐 ℎ𝑎𝑟𝑔𝑒 or 𝑃𝑏𝑎𝑡𝑡𝑒𝑟𝑦 −𝑑𝑖𝑠𝑐 ℎ𝑎𝑟𝑔𝑒 ) to adjust the battery SOC and voltage. It also considers battery degradation models and ensures that the battery power stays within predefined limits.
In the simulation, the PV system was connected to the grid via the boost and 3Ph inverters that were used to supply the AC load. A battery was also used to supply the required power if the PV-generated power was insufficient and store the excess power generated. As seen in Fig. 4, MATLAB Simulink was used to implement the hybrid system. The capacities of the battery-PV system were 205 and 150 W, respectively, while the loads were connected parallel at 100 and 150 kW.
In this mode: the load demanded was assumed to be constant and close to 100 kW while the irradiation, which was supplied at 25 °C, changed from 1000 to 500 W/m2 in 1 s. The variable irradiance decreased the amount of PV power generated from 200 to 100 kW in 1 s and the battery discharged to supply the load demanded after 1 s. The current also remained constant as the load remained constant when the amount of PV power generated changed in relation to changes in irradiation.
Fig. 5(a) depicts the THD of the 3Ph voltage and current. As seen, this began at 0.6 s as three cycles at a frequency of 50 Hz and caused a THD of 3.7%. The battery satisfied the EM requirements by continuously supplying to the load demanded. However, as seen in Fig. 5(b), there was a delay between switching from the PV-generated power to the battery to supply the load demanded. Nevertheless, the system was able to ensure a balance of power between the total amount of power generated by the inverter, the ES system, and the constant Fig 3. The EM algorithm.load voltage and current while keeping the power smooth and within an acceptable THD distortion range. Therefore, the battery system failed to supply the load demanded. In this mode: from time 0–0.12 s, the PV power fails to supply power, so the battery will do so for this short time until the PV generates the power. From time 0.12–1 s PV power is higher load power. Thus, the battery is in a charging state (the generated power is higher than the load power and then the battery observes the current to charge itself). From time 1–2 s, PV power is equal to load power (PV operates in MPPT). So from time 1–2 s, the battery operates in idle mood because the generated power is equal to the load power, so the battery is neither charging nor discharging.
The conditions were the same as those of the previous mode except that the demanded load was close to 250 kW. The amount of PV power generated changed from 200 to 100 kW in 1 s. Fig. 6(a) depicts the total amount of power generated by the battery-PV hybrid system.
In this mode: between 0 and 0.12 s, the PV cells failed to supply the load demanded and the battery supplied it between 0.12 and 1 s, until the PV cells generated the load demanded. Between 0.12 and 1 s, the amount of generated PV power was less than the load demanded. As such, the battery was in discharging state. Between 1 and 2 s, the amount of generated PV power was still less than the load demanded. As such, the battery remained in the discharging state between 1 and 2 s as the amount of generated PV power was insufficient to meet the load demands.
The battery remained in the discharging state throughout the specified time. This mode is dangerous for the system as the battery may run out if the duration is long. Therefore, the system should be equipped with a backup system to address such scenarios. This, however, is not discussed in this present study.
Off-grid self-consumption (OGSC) off-grid hybrid battery-PV system uses self-consumption to ensure continuous power supply [20] [Fig. 6(b)]. This approach utilises a battery to store the excess energy generated by a PV system after the load demanded has been fulfilled. The excess energy is stored in the battery until it reaches its maximum capacity, which is an efficient use of the generated power.
In this mode: the irradiance was assumed to be constant and supplied at 25 °C and 1000 W/m2 while the load demanded changed from 100 to 250 kW in 1 s. Fig. 6(b) provides the amount of power generated by PV cells. The voltage and current were 656.1 V and 312.9 A, respectively. The DC-bus voltage remained constant throughout the mode. The voltage remained constant during the disturbance in the load but the current increased as the load increased when the PV power was constant to 200 kW. Fig. 7(a) depicts THD of the 3Ph voltage and current waveforms. As seen at 0.6 s, the three cycles at a frequency of 50 Hz caused a THD of 4.38% while that at 0.8 s caused a THD of 3.48%.
In this mode: between 0 and 1 s, the amount of PV power generated exceeded the load demanded. As such, the battery was in a charging state. Between 1 and 2 s, the amount of PV power generated was less than the load demanded. At this stage, the PV system entered a mode called the PV-curtail operation, which is when the battery SOC reaches or exceeds its upper limit. The battery discharges power to make up for the difference and supply the balance load when the PV cells fail to generate sufficient power to meet the load demanded. The PV system works at its MPP when it does not produce sufficient power to satisfy the load demanded but the battery SOC remains within its operating threshold. Failures, however, may occur when the hybrid battery-PV system is unable to fulfil the load demanded. As seen, the load demanded was less than the amount of PV power generated until 1 s. The battery discharged and supplied the load demanded in the few instances of delays in PV power generation. When the PV cells resumed supplying power, the battery began charging itself [Fig. 7(b)].
The conditions were the same as those of the previous mode except that the irradiance was decreased to 500 W/m2 and the PV cells generated a constant 100 kW of power. Fig. 8 depicts the battery, PV cells, and hybrid system power generation. As the amount of PV power generated was equal to the load demanded, the battery was in an idle state. Between 1 and 2 s, the amount of PV power generated was less than the load demanded. As such, the battery was neither charging or discharging between 0 and 1 s as the amount of PV power generated was equal to the load demanded. However, between 1 and 2 s, the load demanded exceeded the amount of PV power generated. As such, the battery discharged power to make up for the difference and supply the balance load. The battery can continue doing this as long as its reserves are not completely depleted. This enables the hybrid systems to provide the load demanded, independent of the grid and even when the PV cells fail to produce sufficient power to meet the load demanded by placing the battery in the discharged state.
In this mode: the load demanded was assumed to be constant and close to 100 kW while the irradiance supplied at 25 °C decreased from 1000 to 500 W/m2 in 1 s. Fig. 9(a) shows the PV-generated power profile curve for the whole period. The decrease in irradiance decreased the amount of PV power generated from 200 to 100 kW in 1 s and the battery discharged to supply the load demanded after 1 s. In this mode: between 0 and 1 s, the amount of PV power generated exceeded the load demanded. As such, the battery was in a charging state. If the battery SOC remains within its specified operating limit and there is an excess of power from the PV cells, the PV system works. The excess PV power generated is utilised to charge the battery and ensures that it remains within the desired SOC range. Between 1 and 2 s, the amount of PV power generated was equal to the load demanded. As such, the PV operated at its MPP and the battery was in an idle state as the load demanded was equal to the amount of PV power generated which prevents the battery from charging or discharging. Fig. 9(b) depicts the THD of the 3Ph voltage and current waveforms. As seen at 0.6 s, the three cycles at a frequency of 50 Hz caused a THD of 4.16% while that at 0.8 s caused a THD of 4.56%.
In this mode: the load was assumed to be constant and close to 250 kW while the irradiance supplied at 25 °C changed from 1000 to 500 W/m2 in 1 s. Fig. 10(a) shows the PV-generated power profile curve for the whole period. The decrease in irradiance decreased the amount of PV power generated from 200 to 100 kW in 1 s and the battery discharged to supply the load demanded after 1 s. In this mode: Between 0 and 1 s, the amount of PV power generated was less than the load demanded. As such, the battery was in a discharging state between 1 and 2 s, the amount of PV power generated was less than the load demanded, with the load increasing faster than the PV cells can generate power. Therefore, hybrid systems offer crucial load independence from the grid as the battery will make up the difference, if it is not completely depleted, if the PV cells are unable to generate sufficient power to meet the load demanded. Fig. 10(b) presents the voltage and current results and the THD with an LC filter.
This study explores a dynamic behaviour of PV systems in grid-tied configurations under various operating conditions. It investigates six distinct modes, each characterised by different load demands, PV generation profiles, and battery utilization. Furthermore, the analysis delves into power balance and quality, ensuring that the grid-tied system operates efficiently.
Both modes 1 and 2 experienced changes in PV-generated power from 200 kW to 100 kW in 1 s. In mode 1, the load demanded was 100 kW while in mode 2, it was 250 kW. Battery power was used to compensate for PV delay and ensures that the load was continuously supplied. In mode 2, the battery discharged to make up for the power shortage during the PV delay. Its role also differed based on the load and PV characteristics of the two modes.
Both modes 3 and 4 experienced changes in load demanded from 100 kW to 250 kW in 1 s. Mode 3 experi-enced a constant irradiance of 1000 W/m² while it was reduced to 500 W/m² in mode 4. The battery charged in mode 3 when the amount of PV power generated exceeded the load demanded. This ensures a consistent supply of power. Meanwhile, in mode 4, the battery remained idle as the amount of PV power generated was equal to the load demanded and only discharged when the load demanded exceeded the amount of PV power generated.
Modes 5 and 6 experienced constant load demands of 100 to 250 kW, respectively, with the amount of PV power generated changing from 200 to 100 kW in 1 s. Mode 5 utilised the battery to ensure continuous power supply when the amount of PV power generated exceeded the load demanded. In mode 6, the battery discharged to make up the difference in power shortage when the amount of PV power generated was less than the load demanded. Therefore, the role of the battery switched from supplying power to covering power shortages according to the PV output. Table 3 lists the differences between the discussed modes.
In OGSC, the battery stores the excess PV power for later use to maximise energy use and minimise waste. It differs from other modes as the battery behaves according to changes in the load demanded and the amount of PV power generated.
Table 3.
Comparison between modes 1–6.
Model |
Load demand |
PV profile |
Battery utilization |
1 |
100 kW |
Rapid shift 200 kW to 100 kW |
Compensates for PV delays, maintains power supply |
2 |
250 kW |
Rapid shift 200 kW to 100 kW |
Discharge to bridge power deficit during PV delays |
3 |
250 kW |
Load shift 100 kW to 250 kW |
Charge when PV > demand, maintain consistent supply |
4 |
250 kW |
Load shift 100 kW to 250 kW |
Idle when PV = demand, discharge when demand > PV |
5 |
100–250 kW |
Rapid shift 200 kW to 100 kW |
Engage battery for uninterrupted supply when PV > demand |
6 |
100–250 kW |
Rapid shift 200 kW to 100 kW |
Discharge to compensate for power shortages when PV < demand |
As the charging and discharging patterns of the battery changed according to the load demanded, the amount of PV power generated and the conditions of the modes, it evidently played a critical role in maintaining a stable power supply in most modes. Its operation also changed depending on the PV delay, as well as variations in the loads demanded and irradiance supplied. Power balance between inverter generation, ES, and the voltage and current of the loads was achieved in all the modes. The THDs were within acceptable ranges as well; namely, 3.7% to 4.56%, which ensured that the power quality was maintained even under changing conditions.
This present study assessed the performance of a hybrid battery-PV grid-connected system under various power distortions, such as sudden changes in load demanded and rapid fluctuations in irradiation supplied. MATLAB Simulink was used to simulate a 200 kW PV array connected to a 380 V grid and a battery with a 150 kW capacity. The loads were connected in parallel, with power ratings of 100 to 250 kW. The first strategy involved using the MPPT algorithm to control the boost-based UD DC-to-DC converter circuit while other strategies used PWM for the BD DC-to-DC converter of the ES system and the 3Ph inverter. The results demonstrated that boost and 3Ph inverters can be used to successfully integrate PV systems to a grid by supplying the AC load. A battery was also incorporated into the system to overcome power shortages when insufficient power is generated to store excess power. Although this present study provides valuable insights into the behaviours and performance of the hybrid battery-PV systems that are connected to the power grid under different power distortion scenarios, there are several aspects for future research to improve
1. Using advanced control algorithms: future studies should consider exploring sophisticated control algorithms, such as model predictive control (MPC) and artificial intelligence-based techniques to optimise the system operation, as well as improve its response speed, accuracy, and efficiency.
Research concept and design and writing of the article: B.S.M., N.S., and M.A.S. Data analysis and interpretation and critical revision of the article: S.S., H.BH., and S.L.MH. Final approval of article: B.S.M., N.S., and M.A.S.
Dhar, R. K., Merabet, A., Al-Durra, A. & Ghias, A. M. Y. M. Power balance modes and dynamic grid power flow in solar PV and battery storage experimental DC-link microgrid. IEEE Access 8, 219847– 219858 (2020). https://doi.org/10.1109/ACCESS.2020.3042536
Guerrero-Lemus, R., Cañadillas-Ramallo, D., Reindl, T. & Valle- Feijóo, J. M. A simple big data methodology and analysis of the specific yield of all PV power plants in a power system over a long time period. Renew. Sust. Energ. Rev. 107, 123–132 (2019). https://doi.org/10.1016/j.rser.2019.02.033
Saidi, A. S. Impact of large photovoltaic power penetration on the voltage regulation and dynamic performance of the Tunisian power system. Energy Explor. Exploit. 38, 1774–1809 (2020). https://doi.org/10.1177/0144598720940864
Ali, S., Khan, I., Jan, S., & Hafeez, G. An optimization-based power usage scheduling strategy using photovoltaic-battery system for demand-side management in smart grid. Energies 14, 2201 (2021). https://doi.org/10.3390/en14082201
Kumar, K. R. & Kalavathi, M. S. Power management of grid con- nected hybrid PV/wind/battery power system. Int. J. Eng. Technol. 7, 739–743 (2018). https://doi.org/10.14419/ijet.v7i4.28.28353
Yi, Z., Dong, W. & Etemadi, A. H. A unified control and power management scheme for PV-battery-based hybrid microgrids for both grid-connected and islanded modes. IEEE Trans. Smart Grid 9, 5975–5985 (2018). https://doi.org/10.1109/TSG.2017.2700332
Harsh, P. & Das, D. Energy management in microgrid using incentive- based demand response and reconfigured network considering uncertainties in renewable energy sources. Sustain. Energy Technol. Assess. 46, 101225 (2021). https://doi.org/10.1016/j.seta.2021.101225
Joglekar, J. J. Power and Energy Management in Microgrid. in Microgrid Technologies (Eds. Sharmeela, C., Sivaraman, P., Sanjeevikumar, P. & Bo Holm-Nielsen, J.) 25–56 (John Wiley & Sons, Ltd, 2021). https://doi.org/10.1002/9781119710905.ch2
Roy, K. Optimal energy management of micro grid connected system: A hybrid approach. Int. J. Energy Res. 45, 12758–12772 (2021). https://doi.org/10.1002/er.6609
Kermani, M. et al. Intelligent energy management based on SCADA system in a real microgrid for smart building applications. Renew. Energy 171, 1115–1127 (2021).https://doi.org/10.1016/j.renene.2021.03.008
Talebi, P. & Hejri, M. Distributed control of a grid-connected PV- battery system for constant power generation. J. Energy Manag. Technol. 3, 14–29 (2019). https://doi.org/10.22109/jemt.2019.164589.1145
Hossain, M. A., Chakrabortty, R. K., Ryan, M. J. & Pota, H. R. Energy management of community energy storage in grid-connected microgrid under uncertain real-time prices. Sustain. Cities Soc. 66, 102658 (2021). https://doi.org/10.1016/j.scs.2020.102658
Hasankhani, A. & Hakimi, S. M. Stochastic energy management of smart microgrid with intermittent renewable energy resources in electricity market. Energy 219, 119668 (2021). https://doi.org/10.1016/j.energy.2020.119668
Rangu, S. K., Lolla, P. R., Dhenuvakonda, K. R., & Singh, A. R. Recent trends in power management strategies for optimal operation of distributed energy resources in microgrids: A comprehensive review. Int. J. Energy Res. 44, 9889–9911 (2020). https://doi.org/10.1002/er.5649
Anh, H. P. H. & Van Kien, C. Optimal energy management of microgrid using advanced multi-objective particle swarm optimization. Eng. Comput. 37, 2085–2110 (2020). https://doi.org/10.1108/EC-05-2019-0194
Tazvinga, H., Zhu, B. & Xia, X. Optimal power flow management for distributed energy resources with batteries. Energy Convers. Manag. 102, 104–110 (2015). https://doi.org/10.1016/j.enconman.2015.01.015
Kim, H. J., Kim, M. K. & Lee, J. W. A two-stage stochastic p-robust optimal energy trading management in microgrid operation considering uncertainty with hybrid demand response. Int. J. Electr. Power Energy Syst. 124, 106422 (2021). https://doi.org/10.1016/j.ijepes.2020.106422
Karavas, C.-S., Arvanitis, K. G., Kyriakarakos, G., Piromalis, D. D. & Papadakis, G. A novel autonomous PV powered desalination system based on a DC microgrid concept incorporating short-term energy storage. Sol. Energy 159, 947–961 (2018). https://doi.org/10.1016/j.solener.2017.11.057
Marzal, S., González-Medina, R., Salas-Puente, R., Garcerá, G., &Figueres, E. An embedded internet of energy communication platform for the future smart microgrids management. IEEE Internet Things J.6, 7241–7252 (2019). https://doi.org/10.1109/JIOT.2019.2915389
Hamad, A. A., Nassar, M. E., El-Saadany, E. F. & Salama, M. M. A. Optimal configuration of isolated hybrid AC/DC microgrids. IEEE Trans. Smart Grid 10, 2789–2798 (2019). https://doi.org/10.1109/TSG.2018.2810310
Kofinas, P., Dounis, A. I. & Vouros, G. A. Fuzzy Q-learning for multi- agent decentralized energy management in microgrids. Appl. Energy 219, 53–67 (2018). https://doi.org/10.1016/j.apenergy.2018.03.017
Yang, F., Feng, X. & Li, Z. Advanced microgrid energy management system for future sustainable and resilient power grid. IEEE Trans. Ind. Appl. 55, 7251–7260 (2019).https://doi.org/10.1109/TIA.2019.2912133
Luo, F., Ranzi, G., Wang, S. & Dong, Z. Y. Hierarchical energy management system for home microgrids. IEEE Trans. Smart Grid 10, 5536–5546 (2019). https://doi.org/10.1109/TSG.2018.2884323
Liu, J., Zhang, L. & Cao, M. Power Management and Synchroniza- tion Control of Renewable Energy Microgrid Based on STATCOM. In 2014 IEEE Conference and Expo Transportation Electrification Asia-Pacific (ITEC Asia-Pacific) 1–6 (IEEE, 2014). https://doi.org/10.1109/ITEC-AP.2014.6941242
Yang, Y., Ye, Q., Tung, L. J., Greenleaf, M. & Li, H. Integrated size and energy management design of battery storage to enhance grid integration of large-scale PV power plants. IEEE Trans. Ind. Electron. 65, 394–402 (2018). https://doi.org/10.1109/TIE.2017.2721878
Yüksek, G. & Mete, A. N. A P&O based variable step size MPPT algorithm for photovoltaic applications. Gazi Univ. J. Sci. 36, 608– 622 (2023). https://doi.org/10.35378/gujs.1050325
Makdisie, C. J. & Mariam, M. F. Applied Power Electronics: Rectifiers, Choppers, Regulators. in Handbook of Research on New Solutions and Technologies in Electrical Distribution Networks 362–407 (IGI Global, 2020). https://doi.org/10.4018/978-1-7998-1230-2.ch018
Satyanarayana, K. V. V. & Maurya, R. Power Converters for Integration of Electrical Sources to DC Microgrid. in 2022 IEEE International Conference on Power Electronics, Drives and Energy Systems (PEDES) 1–6 (IEEE, 2022). https://doi.org/10.1109/PEDES56012.2022.10080621
Zhang, G., Dai, Y. & Cui, J. Design and Realization of A Bi- directional DC/DC Converter in Photovoltaic Power System. in Proceedings of the 2016 International Forum on Energy, Environment and Sustainable Development 1095–1100 (Atlantis Press, 2016). https://doi.org/10.2991/ifeesd-16.2016.197
Jaga, O. P., Gupta, R., Jena, B. & GhatakChoudhuri, S. Bi- directional DC/DC converters used in interfacing ESSs for RESs and EVs: A review. IETE Tech. Rev. 40, 334–370 (2023).